Improving Radiographic Fracture Recognition Performance and Efficiency Using Artificial Intelligence
Background
Missed fractures are a common cause of diagnostic discrepancy between initial radiographic interpretation and the final read by board-certified radiologists.
Purpose
To assess the effect of assistance by artificial intelligence (AI) on diagnostic performances of physicians for fractures on radiographs.
Materials and Methods
This retrospective diagnostic study used the multi-reader, multi-case methodology based on an external multicenter data set of 480 examinations with at least 60 examinations per body region (foot and ankle, knee and leg, hip and pelvis, hand and wrist, elbow and arm, shoulder and clavicle, rib cage, and thoracolumbar spine) between July 2020 and January 2021. Fracture prevalence was set at 50%. The ground truth was determined by two musculoskeletal radiologists, with discrepancies solved by a third. Twenty-four readers (radiologists, orthopedists, emergency physicians, physician assistants, rheumatologists, family physicians) were presented the whole validation data set (n = 480), with and without AI assistance, with a 1-month minimum washout period. The primary analysis had to demonstrate superiority of sensitivity per patient and the noninferiority of specificity per patient at –3% margin with AI aid. Stand-alone AI performance was also assessed using receiver operating characteristic curves.
Results
A total of 480 patients were included (mean age, 59 years ± 16 [standard deviation]; 327 women). The sensitivity per patient was 10.4% higher (95% CI: 6.9, 13.9; P < .001 for superiority) with AI aid (4331 of 5760 readings, 75.2%) than without AI (3732 of 5760 readings, 64.8%). The specificity per patient with AI aid (5504 of 5760 readings, 95.6%) was noninferior to that without AI aid (5217 of 5760 readings, 90.6%), with a difference of +5.0% (95% CI: +2.0, +8.0; P = .001 for noninferiority). AI shortened the average reading time by 6.3 seconds per examination (95% CI: –12.5, –0.1; P = .046). The sensitivity by patient gain was significant in all regions (+8.0% to +16.2%; P < .05) but shoulder and clavicle and spine (+4.2% and +2.6%; P = .12 and .52).
Conclusion
AI assistance improved the sensitivity and may even improve the specificity of fracture detection by radiologists and nonradiologists, without lengthening reading time.
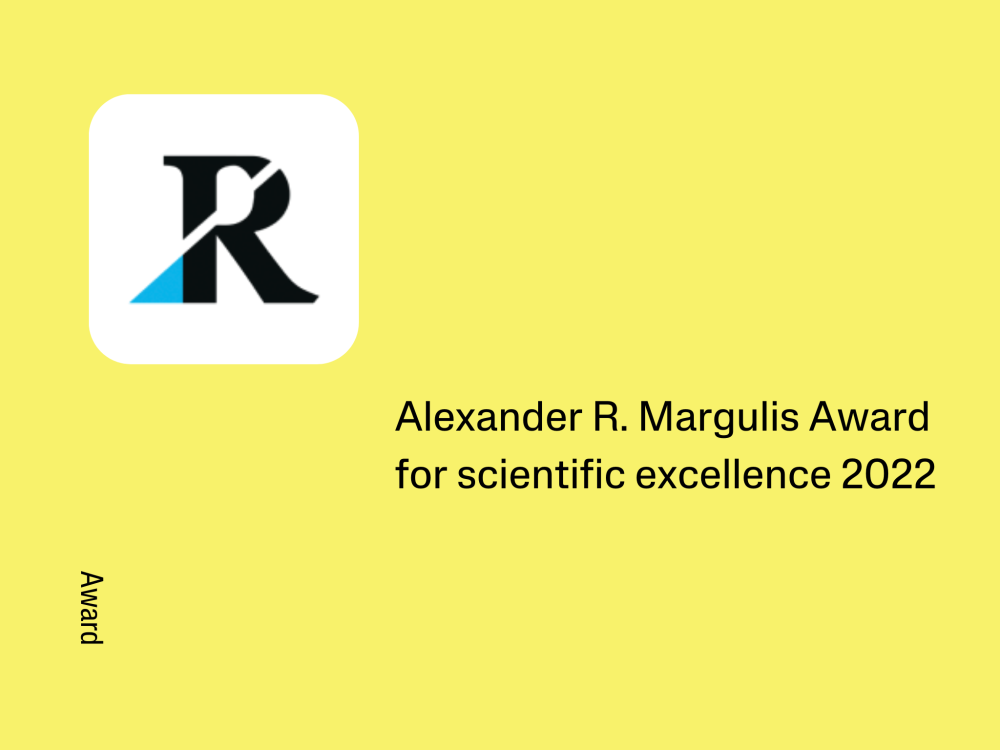
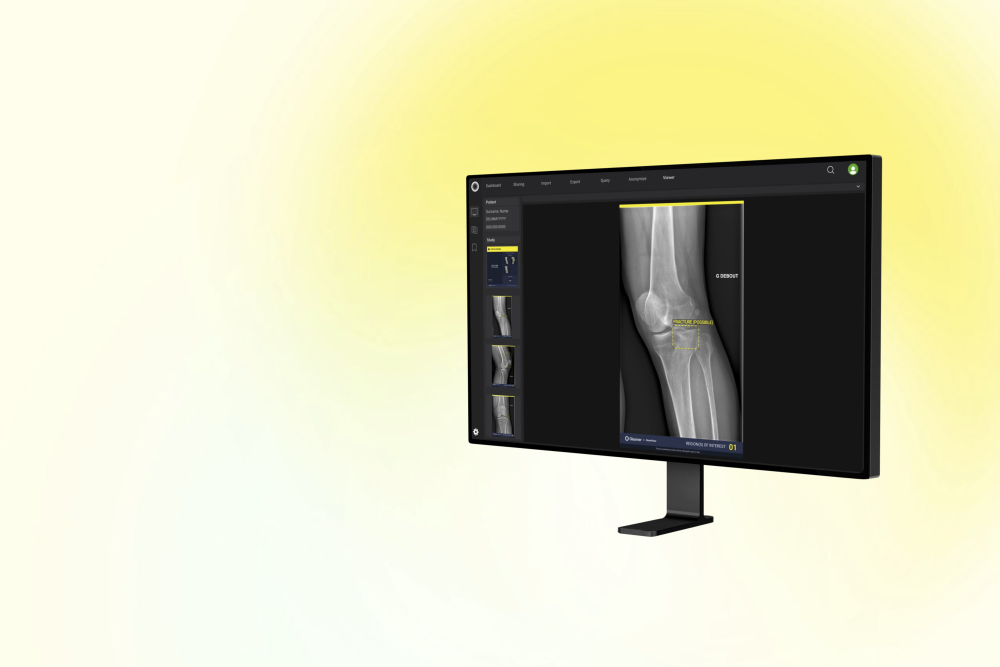